In 2011, NASA, the European Space Agency and the Royal Astronomical Society publicized an open public challenge to be able to map better the small (extremely small) distortion which dark matter creates in our photographs of galaxies. Within the space of one week, a doctoral student specialized in glaciology (natural phenomena caused by water in solid state), came up with an algorithm which was much better than all the astronomic models used until then to map dark matter. In a question of days, he managed to outdo all the work that had been done over the last 10 years.
Where do these kind of people come from, or, better said, where can we find them? People with a privileged capacity to resolve problems, stowaways who go unnoticed in what Eggers and MacMillan call the solution economy? In this case, we can find them in Kaggle, a competition platform in the world of data analysis and design of predictive models.
In exchange for a fee, Kaggle introduces an incentive for mutual advantage in the gap between those who need better analysis and those who have the adequate skills. The essence of this company based in San Francisco is competition, “solutionism”. It is a platform which hosts competitions between statisticians, mathematicians, computer specialists, economists, scientists and anyone with a high analytical profile who is able to propose the most accurate solution for a certain problem. What is ultimately sought? Finding the perfect predictive model.
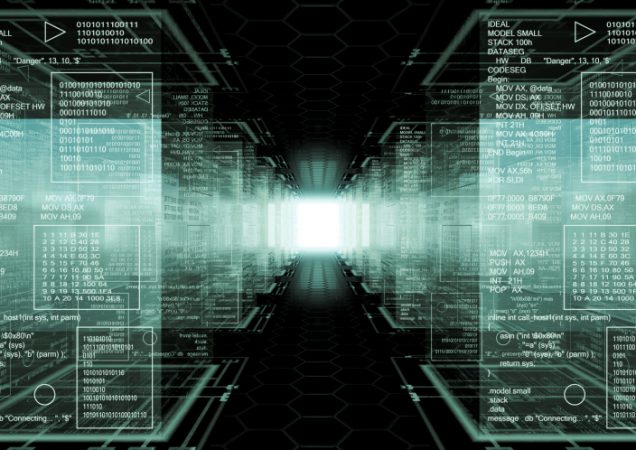
In addition to the payment for results (the glaciologist in question won a reward of 3,000 dollars), Kaggle’s model also includes the exchanging of public value, the payment in the valuable currency of reputation. On the website, a ranking similar to that of the ATP ranking for tennis players or that of the golfing world’s OWGR, shows the score of the best data analysts. In fact, we will find a Spanish participant in this ranking. He currently stands in the tenth position, but he knows what it is like to be right at the top. Therefore, it is logical for many companies in the field of big data to analyze these competitions or who put out adverts to be able to find data analysts with excellent resolution capacity from over 200,000 participants. One of them is Tim Salimans, a Dutch student whose career changed course when he received a Microsoft Research grant for the originality and precision of his predictive models applied to chess. Since then, Salimans has taken part in 14 competitions, and is commonly to be found in the top positions.
For a data scientists (which is the most widely accepted term in English), it is not only a question of the prize, but it is also a powerful incentive to think that their models can be applied, useful, and that they might have a measurable impact on a product or business. A company with a wealth of data has an amazing amount of possibilities. For example, AXA, the insurance company, has launched a competition to find an algorithm which could revolutionize its sector: opening up data about 50,000 car trips, it looks for the algorithm which is best able to find the telematic monitoring to identify the driving patterns making each one of us a unique driver, so predicting risks and offering a customized insurance policy in accordance with this information.
What can I do with these data?
Can the early care and hospitalization process be improved by cross-referencing the data at the disposal of insurance companies? Is it better to improve the Microsoft Kinect gesture detection system? What can you contribute in the search for Higgs’ Boson? It is not easy or intuitive to answer these questions because not all the companies know what to do with their data. This is why Kaggle has introduced a new twist: from competing for the best solution to competing to identify the best problem which should be solved. At the same time, in order to diversify its strategy, Kaggle is beginning to introduce its big data analysis in lucrative sectors such as the energy industry, which it offers solutions to help producers generate more while also bringing down their extraction costs.
On other occasions, the challenge is more simple to grasp: a restaurant chain wants to know what factors play a part in making some establishments successful, while others fail; or large department stores such as Macy’s want to know what facts have an impact on sales. These are examples of challenges which we find in another community, that of the CrowdAnalytics.com website, which brings together over 5,000 data scientists from over 50 countries, most of whom have a Ph.D or MBA, and who propose that their models be used to resolve a business’s needs. The tests on all the models are first tested using public and open data, not using the company’s own information. Sometimes the solution knocks on the door in less than 24 hours.
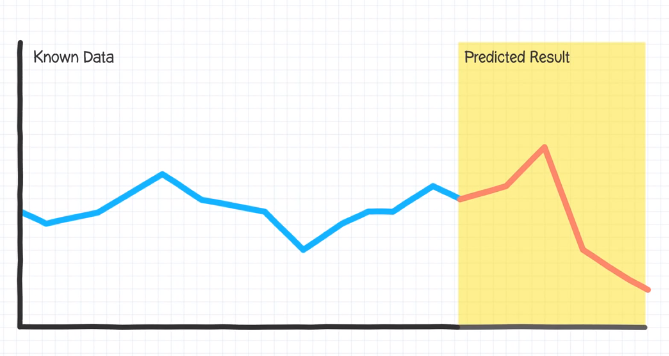
Image: crowdAnalytics.com
Big data for a good cause
The big data environment does not only consist of tech companies, bank product providers, financial services or business intelligence, in fact now more and more figures are emerging from the fields of civil technologies and non-governmental organizations.
DataKind, for example, puts data scientists in contact with third sector organizations to apply their knowledge in humanitarian and social problems. All their work is done for free, and from their base in New York, they coordinate data expert teams in Bangalore, Dublin, San Francisco, Singapore, the UK and Washington. What do they apply their skills to? The help small NGOs to work better on the ground, analyzing social, child-related and educational policies in places such as England and the United States, or, using statistical models applied to legal proceedings, they identify certain patterns followed by judges of the European Court of Human Rights when ruling on different causes. The Economist has coined a term for these geek philanthropists: data huggers.
Bayes impact is working along these same lines, by creating a data model to shorten the response time an ambulance needs to deal with an emergency in the city of San Francisco, and another to understand how a recipient will accept a kidney transplant. It has also collaborated with the Michael J. Fox Foundation by improving systems to diagnose the progress of Parkinson’s disease in patients.
For every solution, every response, every success story in the big data eco-system, a wealth of new questions arise. We have looked at examples of companies, organizations and communities which create new forms of exchange, which perform a type of barter between big data on one hand and the algorithms on the other; reputation in exchange for brilliance and originality. If you don’t know what to do with your data, perhaps you can try to open them in a highly specialized and competitive environment, and see what happens. More and more people are taking the plunge, either favoring collaboration or by encouraging competition, and achieving surprising results.